Maxence Azzouz-Thuderoz is a data scientist specialising in AI and natural language processing at French consulting firm Axys Consultants. Here is how he went from studying economics and econometrics to embracing data science, and why he is now thinking of pursuing a PhD in automatic speech recognition.
Interested in more AI and data science tips? Here’s how to unlock the power of data through a career in data science.
Your background is in economics. How did you end up working as a data scientist?
I went into economics and econometrics because I wanted to be an economist when I was younger. But at the end of my graduate education, I discovered data science.
I got some short lessons and, in the last year of my studies, I decided to change my plans and move to data science.
That’s how I started with economics and ended with a data scientist job.
What did the shift to data science require of you, how was the process?
It requires coding. In economics we didn’t study code that much, so I learned coding. But the transition was kind of easy because I had the mathematical and statistical background that we have in economics and specifically in econometrics, a domain of statistics and economics.
And the transition to data science and traditional machine learning tools was kind of easy because it is about the same mathematical tools, the same statistical tool. So, it was kind of easy.
What was the most challenging part then?
To work with real data. You can find open-source data that is very clean, very nice for studying something, but when you are working with real-world data it can be very complicated.
So, how did you actually manage to get from your economic studies to your current position as a data scientist?
I got a very good friend that found a job in a consulting company in northern France, and he knew that I was looking for an internship. So, he called me and said “Max, I have an opportunity for an internship as a data analyst that I think could be a nice first step for you.”
So, I started at the of commerce with a little internship for my studies and then, when I finished my studies, I went for an internship as a data analyst. Then I found a data scientist job in the consulting industry.
What’s a typical day like in your current role as a data scientist?
Our days are very different because we are always making different things, but, in general, the data scientist job is 70% working with data and 30% is about modelling. No, actually, I would say it’s 70/20 and then maybe 10% is for industrialization. That’s something we have to consider.
What’s the hardest part of working with data these days?
It’s about the information you have about the data.
Some time ago, I was working with a big French banking group, and we did not have all the documentation about the data. Documentation is a very important aspect because you can have the data, but, if you don’t know what it corresponds to, how to work with it, that is a big problem. Sometimes we were working with data that we didn’t completely understand, so we didn’t really understand what we were doing.
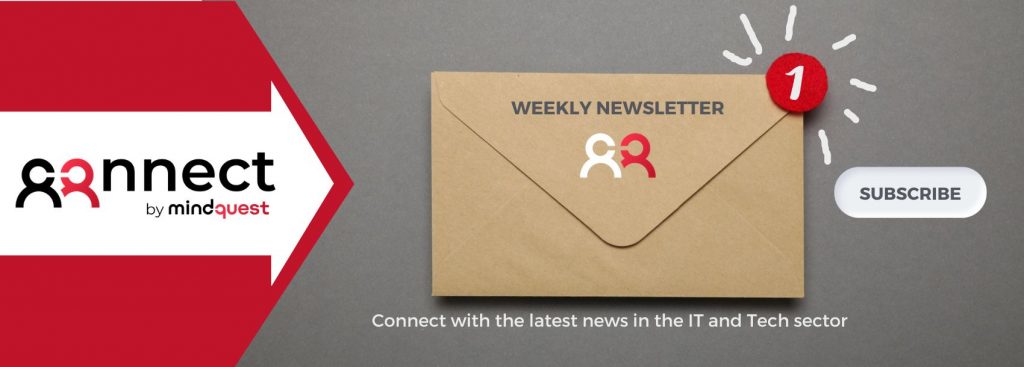
It’s about the information you have about the data.
Some time ago, I was working with a big French banking group, and we did not have all the documentation about the data. Documentation is a very important aspect because you can have the data, but, if you don’t know what it corresponds to, how to work with it, that is a big problem. Sometimes we were working with data that we didn’t completely understand, so we didn’t really understand what we were doing.
Where do you see data science and AI 5 years from now?
I think we will see the first industrial applications of quantum computing in AI.
Additionally, 5G is going to change many things. We will have super-connected houses, apartments, etc. So maybe we will have new projects for AI.
Also, cell phones are becoming more powerful every year. So, I would not be surprised if we have AI technology that today we cannot make work on our phones become a reality in coming years.
For example, automatic speech recognition needs a lot of resources. It’s a big challenge. A big part of current research in automatic speech recognition is about the reduction of the parameters in models so that it all needs fewer resources. So, in the years to come, we might see some kind of automatic speech recognition technology working on smartphones, for instance.
You have decided to pursue a PhD. Why is that?
In my current job, I discovered what it was like to work on research and development projects. It was the first time I was working on such ambitious projects, and I found it very interesting. And I really became aware of the difference in understanding between someone who is simply a skilled data scientist and the scientists, PhD people.
People with a PhD were working on the same project as me, and we were absolutely not at the same level of understanding. I think this is one of the reasons why I want to go ahead with a PhD program. Because I want to reach another level.
And the other reason is that I’ve always been interested in research, the university research system. So yes, it’s a little dream, an old dream that I have and that I think could be nice to realise soon.
So, I recently started to check out universities, looking for a PhD program around automatic speech recognition. It’s a big area.
For more tips on data science, AI and automatic speech recognition, make sure to follow Maxcence on LinkedIn.