We chat with Ton Badal, machine learning engineer at London-based DataOps start-up Synthesized; about pursuing a career in data science and the challenges of working with data.
Check out more of our interviews from our podcast episodes.
How did you get started in tech, what made you go for data science career and machine learning in particular?
Since school, I have had an engineering mentality, I’ve always had this problem-solving way of thinking. I’ve always enjoyed math and solving problems. In university, I studied telecommunications engineering and specialised in audiovisual systems, so the processing of audio, images, video and other audiovisual systems from a technical perspective.
There I started doing research in machine learning, AI and data science. I started discovering this super interesting world. After that, I was sure that I wanted to do a data science career. So I went for a master’s in AI. And that’s how I discovered this very, very interesting and challenging world.
What did you find to be the most challenging part of this process of learning data science as career and becoming a machine learning engineer?
When I started university, it was not a clear path yet. Eighteen or fifteen years ago, you couldn’t see the path of a data scientist from start to end. Data science sits between computer science and math. And, throughout my career, I’ve been closer to computer science than to math. But the challenge is that you have to know as much as possible from both worlds. But at the same time combine them as well as possible. So I think it’s been quite challenging to be able to unify both worlds.
What’s the best career advice you have ever been given?
This is not really a piece of advice that someone has given me, but rather something that I’ve seen people do. I’ve realised that, when I was starting to look for jobs and was looking for a career, I was kind of looking for anything. I felt like I was the only one selling myself. But at some point, you realise that it’s important that the company also sells itself to you. The company also has to be interested in the person who’s applying. It’s not just top-down, but also bottom-up. There has to be this mutual understanding. When I started looking for jobs, I didn’t care that much about that. But after a while, I realised that it’s really important to feel confident and be in a good environment. It’s crucial for your career development and for example a data science career.
So, I would recommend to everyone to not just get the first job and be very selective about what they want and what they seek to accomplish. Also, the people who interview you: you have to look at them and ask as many questions as you can about the company. It’s not only about selling yourself, but also about understanding the company and making sure that the step you’re going to take is the best one for you because that’s going to influence the rest of your career.
CV Writing Tips for IT Professionals
What’s your advice for those who want to learn data science?
If you want to learn something, the best way to learn it is to get hands-on, to find a project that you’re interested in. There are a lot of open source projects that require some help. For example, at Synthesized, we’re now going to open source a fairness package. If you’re interested in this field, you can collaborate on many, many different projects. The best way to learn computer science and data science is to get a project, get a data set. Sign up for a Kaggle Competition, for example, and try to solve it and get as close as you can to the top of the ranking.
Need tips on how to find a job in IT? Check out our IT job hunting guide.
What are the biggest issues with working with data these days?
First of all, there is the problem of ending up with a poor signal-to-noise ratio. The amount of data that you can find nowadays is huge. But, many times, this data contains a lot of noise. And, if you are not careful, you are just going to end up with just a lot of noise that renders it useless.
The second big issue is compliance, so GDPR, HIPAA, etc. If you have data that is not privacy-compliant or that is discriminating against some groups, that’s going to be not only useless, but it’s also going to be illegal to use. So you need to work closely with compliance teams. You need to spend time with the legal team to make sure that you make proper use of your data.
Finally, there’s the problem of data sets becoming data silos. More and more, to access data, you need a data engineer, a data scientist or a machine learning engineer — someone who can do the magic with the data. It’s getting more and more complex to access the data because doing so requires the knowledge of a data engineer or a test engineer.
How is Synthesized helping to solve these problems?
Synthesized has a core engine that is able to solve these problems by enabling users to easily access their data products in many different ways. So, for example, let’s take one of the problems that I was mentioning before: working with compliance and privacy. Our engine is able to generate data that is representative of the original data but is free from privacy issues and from even biases.
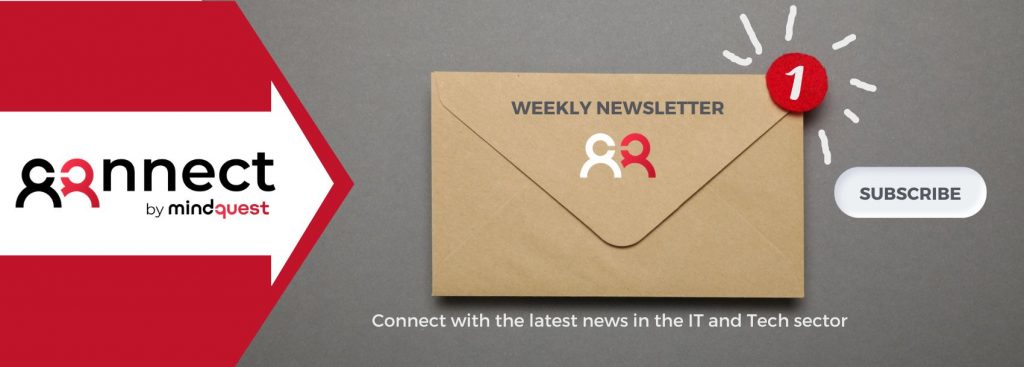
Another of the problems is related to infrastructure, to data silos. Current approaches are data warehouses and data lakes. There are some problems with these approaches, for example, the signal-to-noise ratio in the case of data lakes. There’s a lot of data in there, but it’s very difficult to use. But, the infrastructure problem is also there because the data is very centralised and you need a data engineering team to get to it. So what we’re working on is a new infrastructure called data mesh that aims to decentralise data access. It tries to decentralise all these data products so that each team can access the data independently. Both for internal and for external collaboration.
Can you tell us a bit more about your role at the company?
I’m very lucky to have been a very early employee of the company. I joined at a very early stage, and this meant that, although my official title is machine learning engineer, I’ve been able to touch a bit of everything.
However, my main role as a machine learning engineer is making sure that the core technology is as good as possible. But that also involves a lot of what a pre-sales person would do. So, going to the clients, asking them for requirements, and making sure that the product works well for them and is as tailored as possible to their requirements. But about also improving the product.
And there is also some marketing work involved, like developer relationships. We need to push into that direction because we’re a small company with very new technology and we need to make sure that we sell bottom-up, not top-to-bottom. We approach customers as machine learning engineers, as the nerds who sell to other developers, not as the marketing guys who are trying to sell something to them. Otherwise,+j the message doesn’t get through that well.
What’s next for data? Where do you see data science in, let’s say, five years from now?
I think that, right now, we’re in a very crucial moment for data. We are having all these privacy issues, fairness problems, and the users are more and more aware of this. So, we have to make sure that we have the best practices in place, that we make the best that we can with our data but still respect users. It’s going to be a very challenging time.
At Synthesize, we mainly work with structured data, but I think it’s worth mentioning unstructured data. What’s happening with OpenAI, GPT-3 or other generative models — what’s being done is amazing. It’s a very exciting time. I’m very, very excited to see what the next new thing is going to be.
You’ve been based in London for a while. What do you like the most about the London tech scene?
What I like the most about it is that there are a lot of people working on the same topic, and you can very easily meet people doing really interesting things. And that’s one of the most powerful things when you are doing research or trying to improve your product. Just talking to people, understanding their problems and just having a conversation about something that probably you don’t understand and you don’t even know about.
Discussing new tech trends with people at other companies, that can really help. You discover new things and go out of your usual boundaries. London is great for that because there are a lot of meetups. Well, there were before corona. But yeah, you can talk to and meet a lot of people. There’s this big ecosystem where a lot of things are happening and there’s so much to learn. I’m really happy to be living here.
Join our community and find your next job or expert in IT
You can follow Ton on LinkedIn and on Twitter.
Looking for a job in IT? Check out our IT job hunting guide.