The use of Small Data allows companies to make a good interpretation of Big Data, enabling a more human-centric approach to data processing.
In this article, we discuss this new trend by introducing data consumption prediction and the evolution of data processing.
Data consumption
The amount of data consumed worldwide in 2022 was 947 ZB. And it is expected to reach 180 ZB in 2025 according to Statista data.
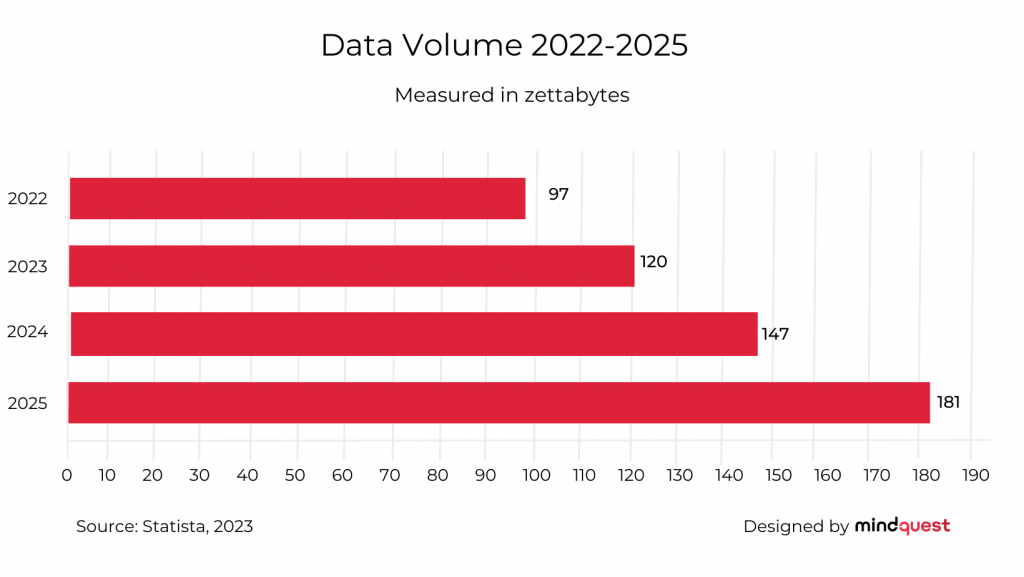
This increase in the use and consumption of data led companies to invest in new technologies. This is to manage and analyze this data in order to gain in-depth knowledge of their customers.
Thus, in 2021, investment in Big Data and data analytics solutions by companies increased by more than 10%. And it is expected that between 2021 and 2025 the annual growth rate will be 12.8%. This investment by companies foresees the business’s need to obtain qualitative information from the data collected.
Despite this, the human aspect of data processing often falls by the wayside in the face of companies’ imperative need to know their customers in depth to achieve business growth.
As a result, companies are beginning to change their metrics to better account for their customers. Thus, as many as 78% of corporate marketing departments have changed their metrics due to the pandemic.
In this sense, Mindquest analyzes how to move from ‘data centric’ to ‘human centric’ in data processing. And this transition involves the introduction of Small Data.
Small Data implementation
Small Data implementation has become critical for companies that want to make profitable use of Big Data. In fact, interpreting Small Data helps companies ensure a service or product that meets real customer needs.
It is no longer just a matter of collecting a large amount of data, but of deriving truly useful information from it.
Following are 3 ways to move from data-centric to human-centric in the data processing
1. Evolution towards Big Data Marketing
The application of data in marketing strategies is a common practice for marketing teams in all companies. Because of this, they have improved their digital strategies.
Thus, companies use Big Data for analytics, but despite its importance in the current context, its collection and analysis is increasingly complicated. This is due to the increased regulation and knowledge of users on the treatment of the same.
For all these reasons, companies must evolve in the treatment of their data and not only take them into account for the benefit of their business, but also to offer real and tangible benefits to their customers and users, taking them into account.
2. Small Data Implementation
Although companies have invested in Big Data in recent years, according to Gartner, by 2025 70 percent of companies will have shifted the focus of their data strategy from Big Data to Small and Wide Data.
“Small data is an approach that involves less data but still provides valuable insights. This approach includes some time-series analysis techniques or few-shot learning, synthetic data or self-supervised learning.” (Gartner, PR May 19, 2021)
The use of small data enables companies to interpret Big Data well, deepening their understanding of customers and their motivations for doing so. They do this by extracting useful information from each customer and opting for data quality rather than quantity.
Its use will be essential in the coming years as companies begin to base their business strategy on the customer. Consequently, they need to know the reasons that motivate their customers’ behavior in order to adapt to them.
The use of small data will enable companies to understand and draw conclusions from the large amount of data they already have on their customers.
3. Be aware of Wide Data
There are more and more data sources or points of contact between a company and its customers. So much so that marketers use data from an average of 15 sources
In this context, Wide Data is essential for companies. This is because it links together data from a wide range of sources to reach a meaningful analysis.
Thus, “Wide data allows analysis and synergy of a variety of small and large, unstructured and structured data sources. It applies X-analytics, where X is looking for links between data sources, as well as for a variety of data formats. These formats include tables, text, images, video, audio, voice, temperature, or even smells and vibrations:” (Gartner, PR May 19, 2021)
Its use allows them to understand customers’ use of each platform and gain a more comprehensive view of them. In this way, companies are able to adjust their strategies accordingly to better engage with their customers.
Conclusion
As important as data is to business strategy, it does not speak for itself.
The entire data analysis team needs to be able to draw conclusions from the data that truly impact the company’s relationship with customers.
In this sense, understanding the work behind data interpretation is essential for each company to enhance the value of its analytics team, which continue to play a determining role in the future of the company.
Also read our article about the differences between Business Intelligence and Big Data. Both work together on data, but they do not do it in the same way. Business Intelligence software helps companies make decisions based on data and metrics. But what does Big Data have to do with it?
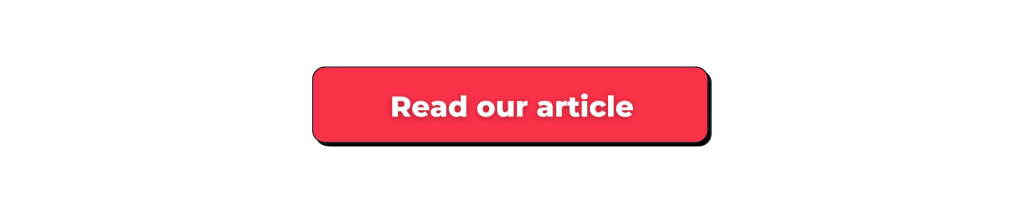