Although they are two closely related concepts, Business Intelligence and Big Data are not the same thing. They are not even interchangeable. In this article, we compare Business Intelligence and Big Data to see their main differences.
Also read our Data Protection Officer Job Description
Differences between Business Intelligence and Big Data
What can we expect from a solution like Business Intelligence (BI) and Big Data? BI or Business Intelligence software helps companies make decisions based on data and metrics. But what does Big Data have to do with it?
Nowadays, there are many companies that use data as a resource. They rely on it to support strategic decisions that help to grow and improve the business. In this aspect, both Big Data and Business Intelligence work together on that data. However, they do not do it in the same way, since we can find differences between them.
But before we look at the differences between Business Intelligence and Big Data, we should give a small definition of both. This will give us enough context to explain their differences.
Also read our Data Center Manager and Big Data Engineer Job Description
What is Big Data?
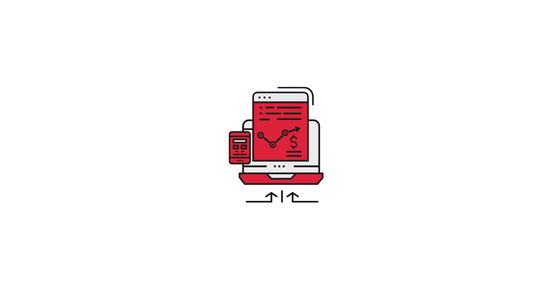
Big Data it is a set of technologies and tools that allow us to manage and process large amounts of data at high speed and in real-time, whether they are structured, semi-structured, or unstructured.
The data come from various sources (smart devices, sensors, social networks, websites, etc.). However, it is not so much the quantity as the quality of the data that matters. In other words, they are usable to generate relevant ideas and make good strategic decisions.
Therefore, when we talk about Big Data, we are referring to a large volume of complex data that is difficult to manage and analyze without the right tools.
Also discover our Database Administrator Job Description
What is Business Intelligence?
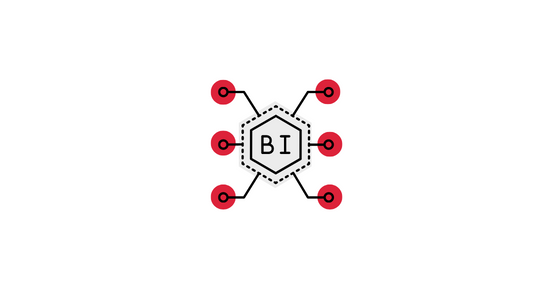
BI is the combination of software applications, infrastructure, and practices that make it possible to access and analyze the information collected by companies. Info they then use to improve decision-making processes.
It is through BI and its tools that we can carry out quality analysis of the data obtained from Big Data.
With Business Intelligence tools, companies can make decisions based on data already processed and treated to convert them into information.
Analyzing the information
The analysis of all this information makes it possible to obtain new data and exploit previously collected information. These processes are useful to:
- Generate new information from the analysis of existing data, e.g. for demand forecasting or people classification methods.
- Identify alarms or exceptional situations to review/study in order to take appropriate action.
The value obtained by these companies translates into:
- Cost savings
- Speed
- New products and service
- The anticipation of the competitors
- Better operational management, etc.
Now that we know what they are, we can see the main differences between Business Intelligence and Big Data.
Also read our Business Intelligence Analyst job description
Main differences between Business Intelligence and Big Data
Comparing these concepts is like talking about two worlds. They are still under exploration, but which constitute the closest reality for companies. Both allow extracting the value of information in totally different but complementary ways.
BI is a set of business management techniques that enable companies to make decisions based on data; Big Data, on the other hand, are the tools that can obtain, store and process data.
In other words:
- Business Intelligence provides access to data sets that are already organized and stored so that the user can easily navigate them,
- Big Data focuses on massive processes for a large volume of data, with very different organization and origins, in order to obtain new information.
This means that with Business Intelligence, companies can carry out analyses and draw conclusions, produce reports, graphs, maps, tables, etc. with 100 per cent detailed information. With Big Data, the opposite is true.
In short, we can summarize the main divergence between the two as ‘innovation and discovery vs. questions and answers‘. Some of the processes that business intelligence uses to deepen data are: the use of software, the feeding of knowledge systems, the transformation of data into actionable intelligence, etc.
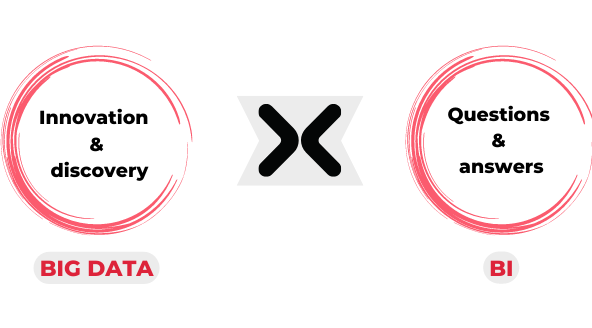
Another difference concerns the type of data with which both methodologies work.
In Big Data all types of data, structured or unstructured, are collected.
Business Intelligence, on the other hand, only works with structured data, which has previously been stored in a database hosted on a server (also called a data warehouse), which allows it to work with offline data.
Business Intelligence and Big Data do not store data in the same way
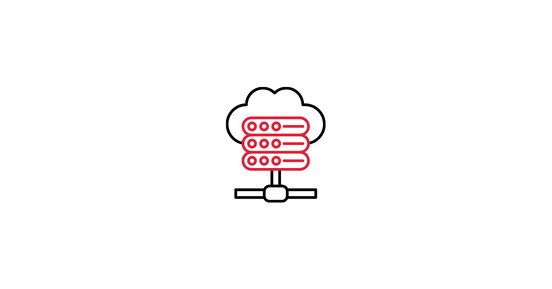
Speaking of data storage; we have already pointed out that Business Intelligence stores data in a database hosted on a server and this must be done prior to processing and analysis.
Big Data, in order to work at the speeds it does, must use several servers to store the large volumes of data, i.e., it must use distributed file systems in nodes to store the information, such as Hadoop. These systems, which are much more flexible (they allow data to be stored without labelling) and more secure, since if one of the nodes fails, the information will be replicated on other nodes.
Data are not processed in the same way
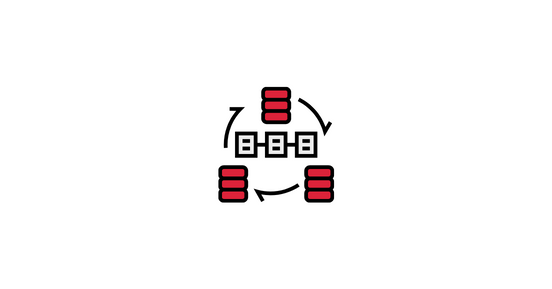
Data processing is not carried out in the same way either.
As we have already mentioned in the previous point, Big Data uses a system of files distributed in nodes, which allows parallel processing of data, thus optimizing the speed at which data is handled. It does this by executing several instructions at the same time, comparing the results obtained, grouping and analyzing them before presenting the final solution to users.
In Business Intelligence, queries must be made to the database to obtain the solutions sought.
Also read our Data Scientist: Job Description
They also differ in how they perform data analysis
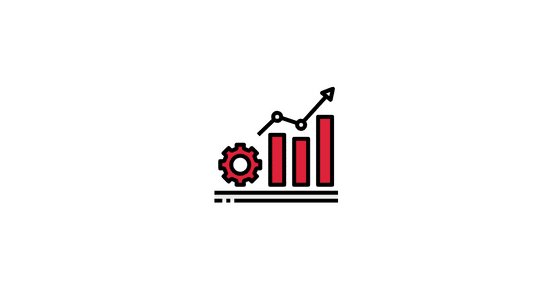
If Big Data can store and process structured and unstructured data, it also has the tools to be able to analyse and visualise large amounts of data, regardless of its type and origin. This is particularly useful for companies, since the vast majority of data currently collected comes from various sources on the Internet and only around 20% is structured.
In addition, Big Data has the ability to work with data from the past as well as in real time, which makes it possible to make more accurate predictions.
Business Intelligence, since it can only work with previously stored, processed, classified and converted data, always works with data from the past.
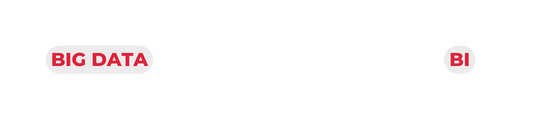
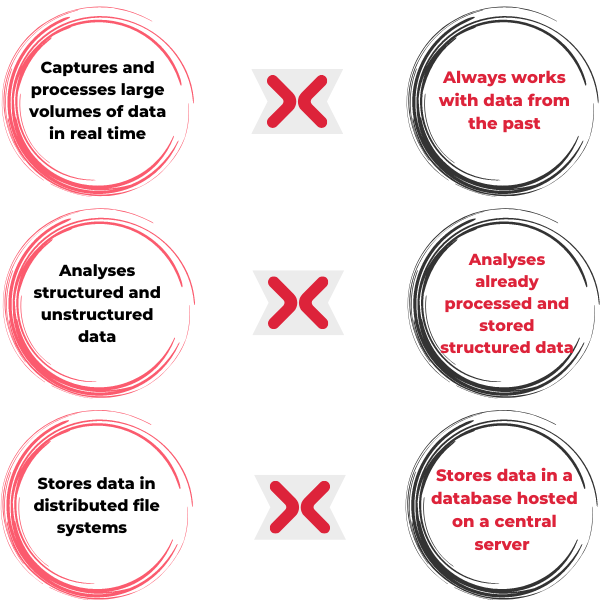
Main differences between Big Data and Business Intelligence
The professional profile is not the same either
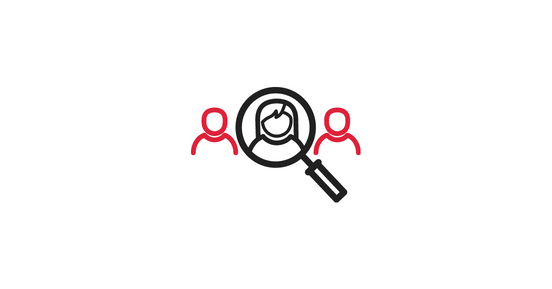
Finally, Big Data and Business Intelligence also differ in the type of professional profile dedicated to each speciality.
On the one hand, the professional profiles for Big Data usually include mathematicians, computer engineers or statisticians. In addition, they belong to the technology department and report to the CTO (Chief Technology Officer).
The data analyst is the leading expert for all business database operations. They assemble and process data in order to evaluate business activity and make appropriate recommendations. Their work enables them to ‘make the data speak’ by interpreting it.
Read the entire job description of the Data Analyst
On the other hand, professional profiles for Business Intelligence come from fields such as business administration, economists or marketing, although they may also include engineers or technicians.
For example, QlikView is a Business Intelligence platform facilitating self-service data interpretation. Thus, the QlikView solution enables big data analysis to be transformed into actionable insights. As a consequence, the role of the QlikView developer is to prepare prior data processing to adapt the tool to the business needs and the activities of the company.
Meanwhile, the role of the IT Business Analyst aims to bridge the gap between the various operational departments and the IT department.
They are usually found in the company’s management department and report either to the CSO (Chief Strategy Officer) if there is one, or directly to the CEO.
Also discover the role of the IoT Consultant
Why Business Intelligence is important
Businesses are undergoing the shift towards digital transformation. More and more businesses are seeing the need to invest in data analytics solutions for one simple reason: information is power.
Through them, we can have full control of data and increase business insight, as well as:
- Save time and costs.
- Improve the service offered to customers.
- Facilitate the consultation of data.
- New business opportunities.
- Obtain verified, transparent and reliable results.
Having exposed these advantages, we can affirm that having a BI platform is essential to achieve business success.
Although each company is a different world, all of them can find competitive advantages in BI. Solutions focused on business intelligence are no longer seen as a simple tool focused only on large companies, so more and more SMEs are becoming interested in its technology.
What you shouldn’t do in Business Intelligence
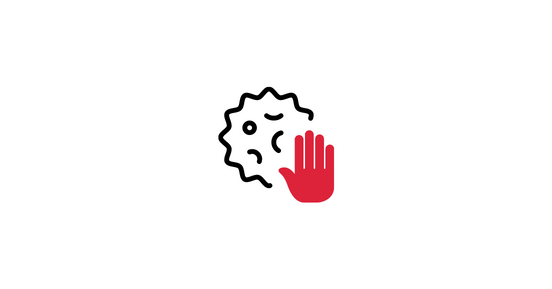
Here are the things you should avoid at all costs when it comes to BI:
- Choosing a technology that does not meet your business requirements, needs, or problems.
- Poorly defined software objectives in the planning phase.
- Forgetting the role of the end user.
- Lack of integration and protection of company data.
- Leaving the back-end in the background and giving top priority to the front-end. They must be in balance.
Learn more about the most common mistakes to avoid in Business Intelligence
So, Big Data vs Business Intelligence, which wins?
The truth is that neither, because it is not a competition between the two methodologies. But rather they must work together to get the most out of data collection and analysis.
Thus, the Business Intelligence team will work together with the Big Data team. They need to establish the data to collect and then go on and analyze it. For its part, the Big Data team will look for patterns in the data to communicate them to the BI team.
Also discover the role of the Artificial Intelligence (AI) expert
The next Business Intelligence challenge: real-time analysis
If BI wants to remain relevant and not be displaced over time by Big Data tools, it must take the next step and be able to have its own tools for real-time data analysis.
In other words, BI will also need to carry out analysis on unstructured data and achieve a system in which it is possible to detect and respond to situations that occur in the market in a quick and agile manner.
This does not mean that Business Intelligence will stop working together with Big Data. This is because the process of collecting and storing massive data will continue to fall to the latter. But it does mean that the former will have tools that allow it to analyze these data in real time. This without having to process, treat and store them in a database as it has been doing until now.
You can also read : 10 Business Intelligence Stats That Show Its Worth
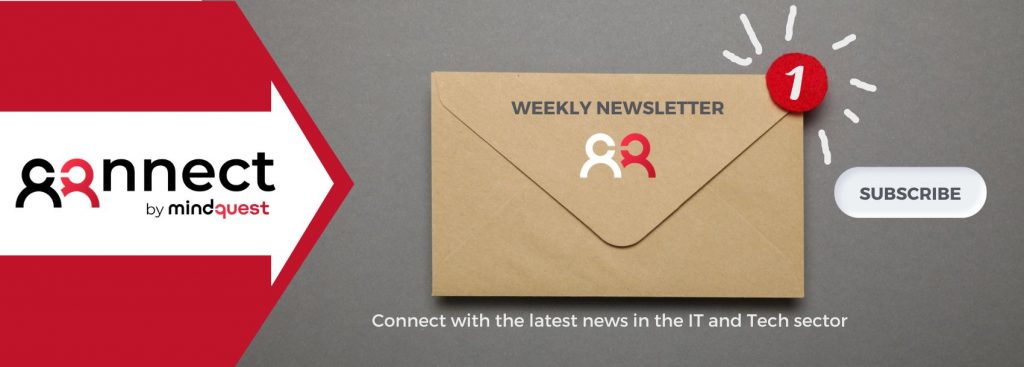